6. Energy Budget#
Definitions#
There are four types of energy (per unit mass) that we need to keep track of in the fluid:
\(I = c_v T\) is the internal energy (valid for an ideal gas, see e.g. Vallis [2017]) – this is a measure of the disorganized molecular-scale motion in the fluid
\(\Phi = g z\) is the gravitational potential energy
\(L = L_v q\) is the latent energy associated with the evaporated water in the air (\(L_v\) is the latent heat of vaporization and \(q\) is the specific humidity)
\(K = \frac{1}{2} \left(u^2 + v^2 + w^2 \right)\) is the kinetic energy of organized motion of the fluid
We’ll define the total energy per unit mass in the atmosphere as
Budget equations for energy components#
Potential energy#
Latent energy#
Internal energy#
Kinetic energy#
A total energy equation and global conservation#
After some work and cancellation of various conversion terms:
with \(D_h = c_p D_T + L_v D_q\) the net surface enthalpy flux and \(\vec{F}_{rad}\) the radiative flux.
Alternate form and Moist Static Energy#
We can rewrite the first law of thermodynamics as
where \(c_p T\) is refered to as the sensible energy \(S\)
Using this, we can define another form of total energy
and we’ll find it useful to define the moist static energy \(m\) as the first three terms of \(mathcal{E}\):
We’ll see from the data that \(K\) is typically a small fraction of \(\mathcal{E}\) so is often ignored in the total energy budget, and we will talk instead about fluxes of moist static energy rather than fluxes of total energy.
Merdional energy transport#
Write the zonal- and time-averaged energy budget in pressure coordinates and flux form:
and we can decompose the meridional energy flux into its components:
Finally we can decompose each component into mean meridional, stationary eddy, and transient eddy terms, e.g.
and likewise for the other components.
The observed energy budget#
import numpy as np
import xarray as xr
import matplotlib.pyplot as plt
cfsr_path = '/cfsr/data/'
year = '2021'
ds = xr.open_mfdataset(cfsr_path + year + '/*.nc', chunks={'time':30, 'lev': 1})
ds
/knight/anaconda_aug22/envs/aug22_env/lib/python3.10/site-packages/dask/array/core.py:4796: PerformanceWarning: Increasing number of chunks by factor of 32
result = blockwise(
/knight/anaconda_aug22/envs/aug22_env/lib/python3.10/site-packages/xarray/core/indexing.py:1374: PerformanceWarning: Slicing is producing a large chunk. To accept the large
chunk and silence this warning, set the option
>>> with dask.config.set(**{'array.slicing.split_large_chunks': False}):
... array[indexer]
To avoid creating the large chunks, set the option
>>> with dask.config.set(**{'array.slicing.split_large_chunks': True}):
... array[indexer]
return self.array[key]
/knight/anaconda_aug22/envs/aug22_env/lib/python3.10/site-packages/dask/array/core.py:4796: PerformanceWarning: Increasing number of chunks by factor of 10
result = blockwise(
/knight/anaconda_aug22/envs/aug22_env/lib/python3.10/site-packages/dask/array/core.py:4796: PerformanceWarning: Increasing number of chunks by factor of 32
result = blockwise(
/knight/anaconda_aug22/envs/aug22_env/lib/python3.10/site-packages/dask/array/core.py:4796: PerformanceWarning: Increasing number of chunks by factor of 32
result = blockwise(
/knight/anaconda_aug22/envs/aug22_env/lib/python3.10/site-packages/xarray/core/indexing.py:1374: PerformanceWarning: Slicing is producing a large chunk. To accept the large
chunk and silence this warning, set the option
>>> with dask.config.set(**{'array.slicing.split_large_chunks': False}):
... array[indexer]
To avoid creating the large chunks, set the option
>>> with dask.config.set(**{'array.slicing.split_large_chunks': True}):
... array[indexer]
return self.array[key]
/knight/anaconda_aug22/envs/aug22_env/lib/python3.10/site-packages/dask/array/core.py:4796: PerformanceWarning: Increasing number of chunks by factor of 32
result = blockwise(
/knight/anaconda_aug22/envs/aug22_env/lib/python3.10/site-packages/dask/array/core.py:4796: PerformanceWarning: Increasing number of chunks by factor of 10
result = blockwise(
/knight/anaconda_aug22/envs/aug22_env/lib/python3.10/site-packages/xarray/core/indexing.py:1374: PerformanceWarning: Slicing is producing a large chunk. To accept the large
chunk and silence this warning, set the option
>>> with dask.config.set(**{'array.slicing.split_large_chunks': False}):
... array[indexer]
To avoid creating the large chunks, set the option
>>> with dask.config.set(**{'array.slicing.split_large_chunks': True}):
... array[indexer]
return self.array[key]
/knight/anaconda_aug22/envs/aug22_env/lib/python3.10/site-packages/dask/array/core.py:4796: PerformanceWarning: Increasing number of chunks by factor of 32
result = blockwise(
/knight/anaconda_aug22/envs/aug22_env/lib/python3.10/site-packages/dask/array/core.py:4796: PerformanceWarning: Increasing number of chunks by factor of 10
result = blockwise(
/knight/anaconda_aug22/envs/aug22_env/lib/python3.10/site-packages/xarray/core/indexing.py:1374: PerformanceWarning: Slicing is producing a large chunk. To accept the large
chunk and silence this warning, set the option
>>> with dask.config.set(**{'array.slicing.split_large_chunks': False}):
... array[indexer]
To avoid creating the large chunks, set the option
>>> with dask.config.set(**{'array.slicing.split_large_chunks': True}):
... array[indexer]
return self.array[key]
/knight/anaconda_aug22/envs/aug22_env/lib/python3.10/site-packages/dask/array/core.py:4796: PerformanceWarning: Increasing number of chunks by factor of 32
result = blockwise(
<xarray.Dataset> Dimensions: (time: 1460, lat: 361, lon: 720, lev: 40) Coordinates: * time (time) datetime64[ns] 2021-01-01 ... 2021-12-31T18:00:00 * lat (lat) float32 -90.0 -89.5 -89.0 -88.5 -88.0 ... 88.5 89.0 89.5 90.0 * lon (lon) float32 -180.0 -179.5 -179.0 -178.5 ... 178.5 179.0 179.5 * lev (lev) float32 -2e-06 2e-06 10.0 20.0 ... 925.0 950.0 975.0 1e+03 Data variables: (12/16) g (time, lev, lat, lon) float32 dask.array<chunksize=(30, 3, 361, 720), meta=np.ndarray> pmsl (time, lat, lon) float32 dask.array<chunksize=(30, 361, 720), meta=np.ndarray> pres_pv (time, lev, lat, lon) float32 dask.array<chunksize=(30, 1, 361, 720), meta=np.ndarray> psfc (time, lat, lon) float32 dask.array<chunksize=(30, 361, 720), meta=np.ndarray> pv_isen (time, lev, lat, lon) float32 dask.array<chunksize=(30, 15, 361, 720), meta=np.ndarray> pwat (time, lat, lon) float32 dask.array<chunksize=(30, 361, 720), meta=np.ndarray> ... ... u_isen (time, lev, lat, lon) float32 dask.array<chunksize=(30, 15, 361, 720), meta=np.ndarray> u_pv (time, lev, lat, lon) float32 dask.array<chunksize=(30, 1, 361, 720), meta=np.ndarray> v (time, lev, lat, lon) float32 dask.array<chunksize=(30, 3, 361, 720), meta=np.ndarray> v_isen (time, lev, lat, lon) float32 dask.array<chunksize=(30, 15, 361, 720), meta=np.ndarray> v_pv (time, lev, lat, lon) float32 dask.array<chunksize=(30, 1, 361, 720), meta=np.ndarray> w (time, lev, lat, lon) float32 dask.array<chunksize=(30, 3, 361, 720), meta=np.ndarray> Attributes: description: g 1000-10 hPa year: 2021 source: http://nomads.ncdc.noaa.gov/data.php?name=access#CFSR-data references: Saha, et. al., (2010) created_by: User: ab473731 creation_date: Sat Jan 2 06:01:03 UTC 2021
def bracket(data):
return data.mean(dim='lon', skipna=True)
def star(data):
return data - bracket(data)
def bar(data, interval=ds.time.dt.month):
return data.groupby(interval).mean(skipna=True)
def prime(data, interval=ds.time.dt.month):
return data.groupby(interval) - bar(data, interval=interval)
def global_mean(data):
return data.weighted(np.cos(np.deg2rad(data.lat))).mean(dim=('lat','lon'), skipna=True)
v = ds.v
T = ds.t - global_mean(ds.t) # we subtract the global mean everywhere to avoid large cancellations
vbar = bar(v)
vbracket = bracket(v)
vbarbracket = bracket(vbar)
Tbar = bar(T)
Tbracket = bracket(T)
Tbarbracket = bracket(Tbar)
vT = bracket(bar(v*T))
vT_transient = bracket(bar(prime(v)*prime(T)))
vT_stationary = bracket(star(vbar) * star(Tbar))
vT_mmc = vbarbracket * bracket(Tbar - global_mean(Tbar))
vT
<xarray.DataArray (month: 12, lev: 40, lat: 361)> dask.array<mean_agg-aggregate, shape=(12, 40, 361), dtype=float32, chunksize=(1, 4, 361), chunktype=numpy.ndarray> Coordinates: * lat (lat) float32 -90.0 -89.5 -89.0 -88.5 -88.0 ... 88.5 89.0 89.5 90.0 * lev (lev) float32 -2e-06 2e-06 10.0 20.0 ... 925.0 950.0 975.0 1e+03 * month (month) int64 1 2 3 4 5 6 7 8 9 10 11 12
flux_list = [vT, vT_transient, vT_stationary, vT_mmc]
levs = np.arange(-50,55,5)
fig, axes = plt.subplots(4,1, figsize=(8,12))
for num, flux in enumerate(flux_list):
flux_jan = flux.sel(month=1)
CS = flux_jan.plot.contour(ax=axes[num],
yincrease=False,
levels=levs,
)
axes[num].clabel(CS)
axes[0].set_title('Total sensible heat flux $[\overline{vT}]$ (January)')
axes[1].set_title('Sensible heat flux by transient eddies $[\overline{v^\prime T^\prime}]$ (January)');
axes[2].set_title('Sensible heat flux by stationary eddies $[\overline{v}^* \overline{T}^*]$ (January)')
axes[3].set_title('Sensible heat flux by MMC $[\overline{v}][\overline{T} - \overline{T}_{global}]$ (January)')
/knight/anaconda_aug22/envs/aug22_env/lib/python3.10/site-packages/flox/aggregations.py:258: RuntimeWarning: invalid value encountered in true_divide
finalize=lambda sum_, count: sum_ / count,
Text(0.5, 1.0, 'Sensible heat flux by MMC $[\\overline{v}][\\overline{T} - \\overline{T}_{global}]$ (January)')
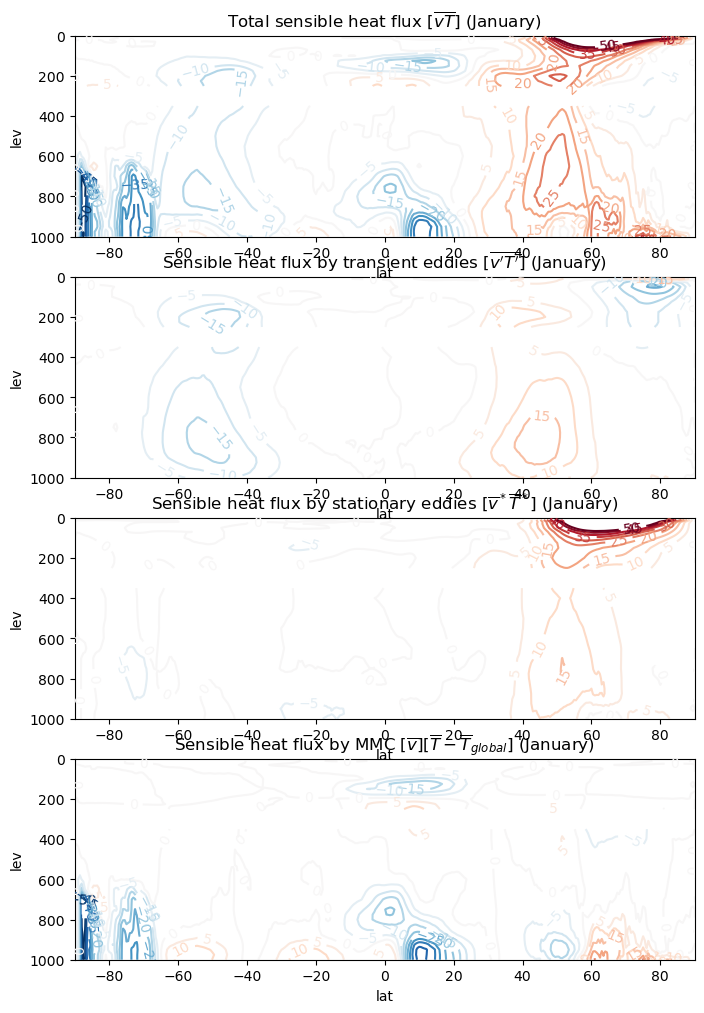